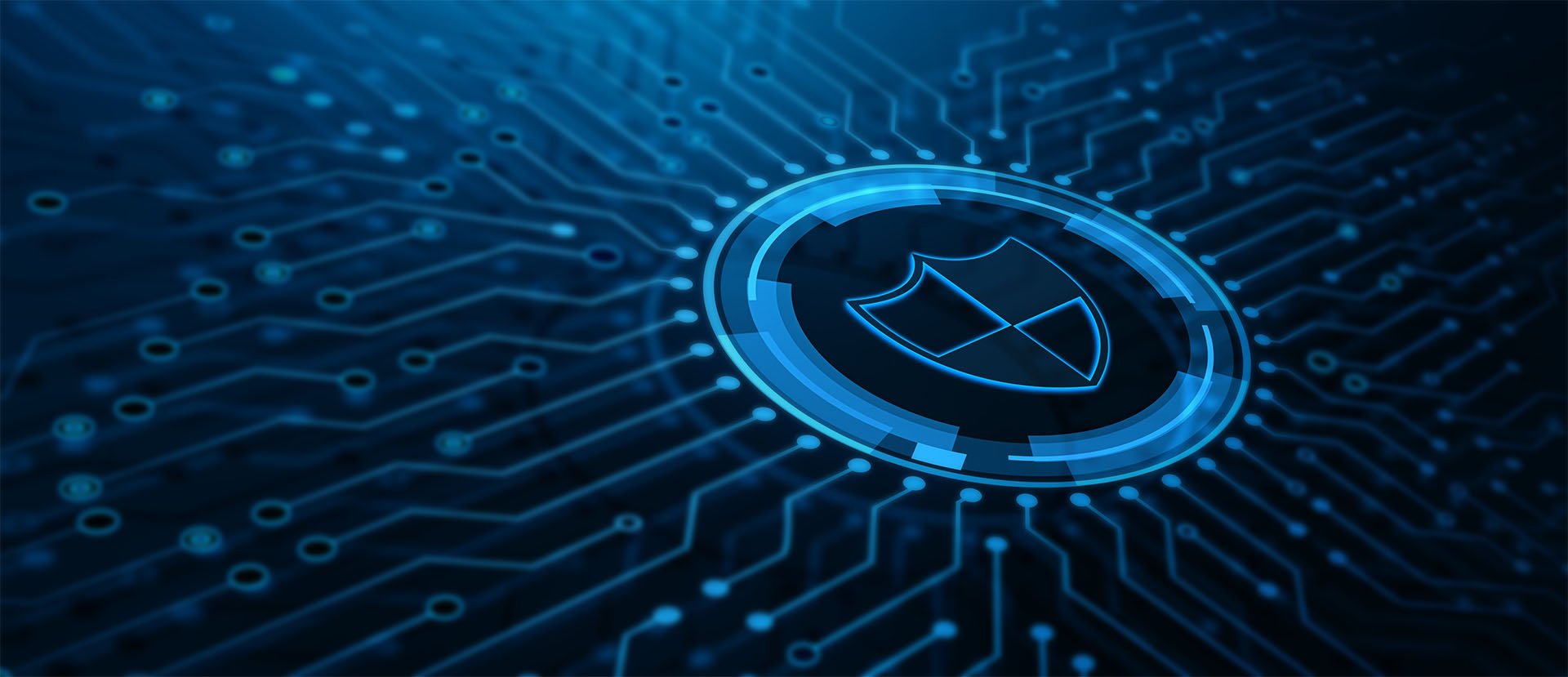
An important part of security is defence in depth: multiple layers of defence used to reduce the probability of a successful attack on a system or organisation. Crucially, for defence in depth these defences must be diverse in their ability to detect and/or prevent intrusion attempts. Here, as in security in general, there is a need to support decisions through quantitative approaches, seeking to answer questions like: "should a given available budget be spent on a specific defence X or two weaker defences Y and Z which, however, if combined promise better security than X alone?", "in this threat environment, what is the likelihood of a successful intrusion achieving damage worth D over one year?" etc. This project aims to produce methods for answering such questions, inevitably in probabilistic terms, with clear understanding of how much trust can be put in these methods. We will consider these layers of defence: AntiVirus (AV) products, Intrusion Detection Systems (IDS), Firewalls, as well as the implicit layers for defence created by the inherent robustness to attack of the applications and platforms being attacked (e.g. diverse operating systems and applications). The probabilistic models that will result from this research will be of two broad types: - Conceptual models - models that are used to conceptualise the problem domain and enable understanding of relative importance of different factors and theoretical limits of the benefits of diversity with the various protection layers but that are defined at a reasonably high level of abstraction making it difficult to observe and quantify the parameters of these models in practice; - Operational models - models the parameters of which can be observed and the model can be used in operation for security assessment and prediction. Successful operation models achieve prediction, given a sequence of previous observations, in the presence of limited change. Successful conceptual models more modestly clarify non-intuitive universal truths and help to analyse scenarios (e.g. showing best- and worst-case effects rather than likely effects) for which data are insufficient for prediction The open problems that we address regarding the assessment of the potential gains from defence in depth include: - Designing multi-layered defences. There are at least three dimensions to the design: - The choice of diversity architecture: how many devices, how many types of devices etc.) - How they are combined (e.g., for products that flag possible attacks, whether a security response requires consensus among multiple layers, or just one to give an alarm,or a certain majority is required). - The nature of the assets to be protected. - Security requirements are usually expressed in terms of (at least) three constituent attributes: Confidentiality, Integrity and Availability (CIA). An important issue is that designs that improve one of these attributes may make others worse, and probabilistic models help to manage these trade-offs. - There is a difference between measuring how secure a defence system has been in the past and predicting how secure it will be, as attackers develop new techniques and security vendors try to adapt. We need methods that allow us to predict the security of one (or several) layers of defences based on what we have seen in the past. Predictions may be in terms of the probabilities of: the time to next attack; the rate of attacks that we can expect in a given time interval; vulnerabilities existing in a set of defences etc; and since these will never be infallible we need methods for assessing how well they perform so that their users know how much confidence to have in these predictions.