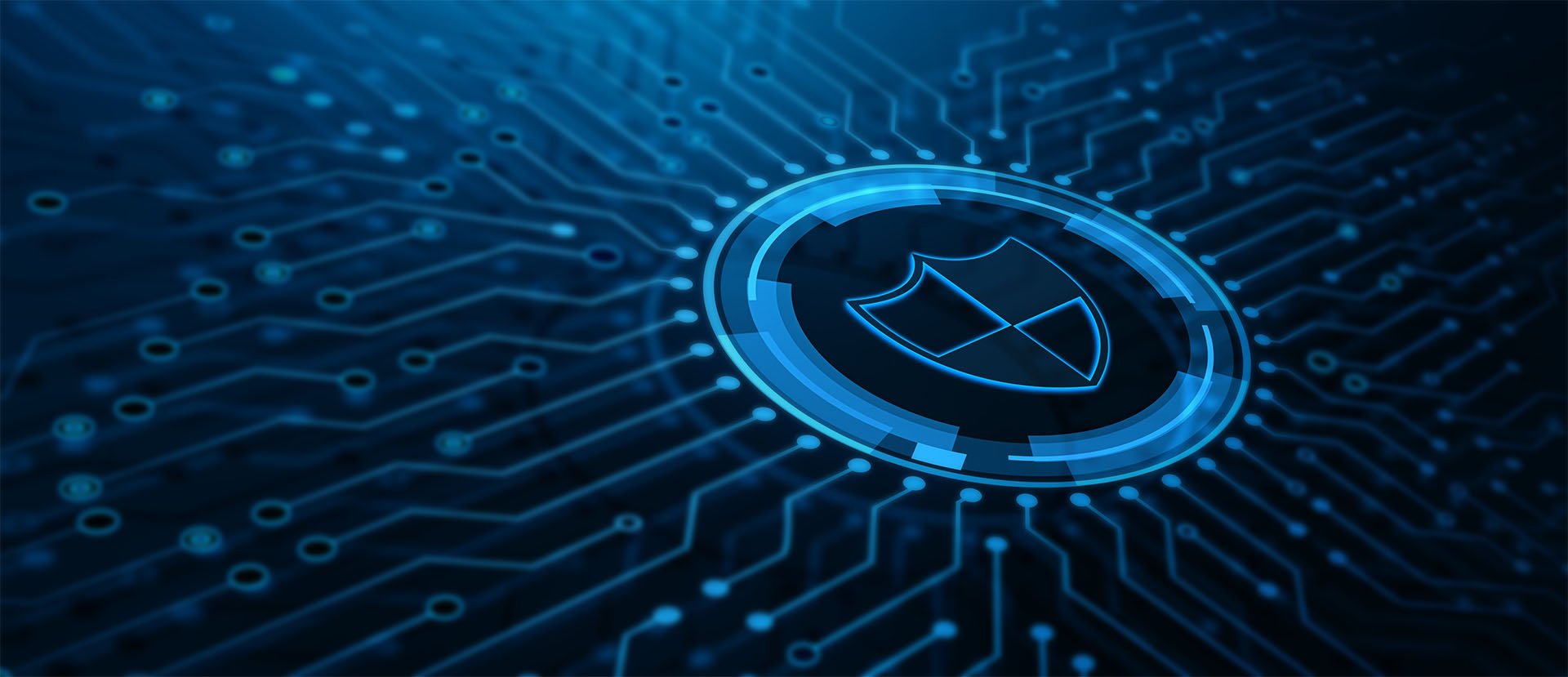
Abstract: In order for a dataset to be legally compliant - in some sense - with privacy laws such as the General Data Protection Regulation (GDPR) various steps must be taken to ensure the removal of data that might compromise or reveal personal data. This can be achieved through a process of removal of information content or semantics; which if done incorrectly can render that dataset in violation of such laws. Machine learning presents a technology based around the analysis of dependencies and correlations of a dataset. This can be used to measure information content within the bounds of the dependencies estimators used. Utilising this we can measure the effects of anonymisation upon a dataset and the efficacy of said anonymisation functions. If we additionally characterise what anonymisation means in terms of information loss and construct classification functions we have a framework in which the decision over whether an anonymisation is sufficient can be made. This can then be extended to an automation scenario where it becomes potentially possible that texts such as as the GDPR can be rendered as said classification functions.
Cyberwatching.eu has received funding from the European Union’s Horizon 2020 research and innovation programme under grant agreement No 740129. The content of this website does not represent the opinion of the European Commission, and the European Commission is not responsible for any use that might be made of such content. Privacy Policy | Disclaimer / Terms and Conditions of Use